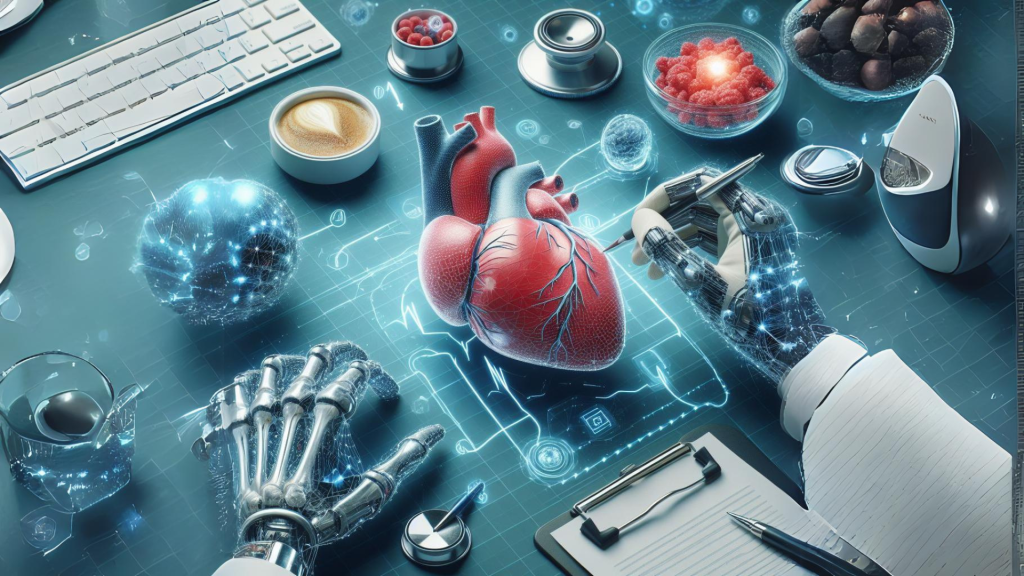
Addressing the challenge of diuretic responsiveness in acute decompensated heart failure (ADHF) patients, researchers at The Texas Heart Institute unveil a novel machine learning (ML) tool aimed at predicting treatment outcomes. With ADHF posing significant risks and complexities in patient management, the development of predictive models like BAN-ADHF marks a crucial advancement in personalized cardiac care.
1. Diuretic Responsiveness in ADHF: A Clinical Conundrum
1.1 Clinical Landscape:
ADHF complications necessitate efficient diuretic therapy for optimal patient outcomes.
Variability in treatment response underscores the need for tailored interventions to mitigate diuretic resistance.
1.2 Challenges and Implications:
Inefficient diuretic response heightens risks of rehospitalization and mortality, warranting early identification and intervention.
Lack of consensus on dosage adjustments complicates treatment strategies, highlighting the need for predictive tools.
2. Introducing BAN-ADHF: ML for Personalized Treatment
2.1 Methodology:
Leveraging de-identified clinical trial data, researchers developed the BAN-ADHF model to predict diuretic responsiveness.
Utilized ML phenomapping to classify patients into subgroups based on diuretic efficiency and treatment outcomes.
2.2 Clinical Utility:
BAN-ADHF accurately identifies patients with varying diuretic responses, enabling tailored treatment approaches.
Predictive capabilities offer prognostic utility, facilitating risk stratification and optimizing clinical decision-making.
3. Clinical Translation and Future Directions
3.1 Implementation Challenges:
Translation of BAN-ADHF into clinical practice requires validation through prospective studies and integration into care protocols.
Evaluation of real-world effectiveness is crucial to assessing impact on patient outcomes and healthcare delivery.
3.2 Broader Implications:
ML-driven tools like BAN-ADHF exemplify the transformative potential of AI in cardiovascular care.
Future research should focus on expanding predictive models to address diverse cardiac conditions and enhance precision medicine approaches.
4. Beyond Heart Failure: AI in Cardiovascular Risk Prediction
4.1 Multifaceted Applications:
ML models extend beyond ADHF to predict adverse outcomes in percutaneous coronary intervention (PCI) patients.
Smartphone and computer applications offer accessible decision support tools for clinicians, enhancing risk assessment and shared decision-making.
4.2 Integration and Collaboration:
Collaboration between clinicians, researchers, and technology developers is vital for advancing AI-driven solutions in cardiovascular medicine.
Continuous refinement and validation are essential to ensure clinical efficacy and widespread adoption of ML tools.
The development of BAN-ADHF underscores the transformative potential of ML in addressing complex cardiac challenges. By harnessing predictive analytics, clinicians can tailor treatment strategies, mitigate risks, and improve patient outcomes in ADHF and beyond. As AI continues to reshape cardiovascular care, interdisciplinary collaboration and evidence-based validation remain paramount for translating innovative solutions into clinical practice, ultimately advancing precision medicine paradigms in cardiac healthcare.